Engineering
Sep 26, 2024
Engineering
Finetuning Domain adaptive language model with FastTrack
Yonggeun Kwon
Research Intern
Sep 26, 2024
Engineering
Finetuning Domain adaptive language model with FastTrack
Yonggeun Kwon
Research Intern
Introduction
This article explains how to train and evaluate a language model specialized in supply chain and trade-related domains using Backend.AI's MLOps platform, FastTrack. For this language model, we used the gemma-2-2b-it model as the base model, which was continually pretrained with supply chain and trade domain datasets. To train a model specialized in the Question Answering task, domain datasets collected and processed from the web were converted into a Q/A task format, consisting of trainable questions and answers, depending on the use case.
Developing AI involves stages such as data preprocessing, training, validation, deployment, and inference. Using Lablup's FastTrack, each of these stages can be configured into a single pipeline, allowing for easy customization, such as skipping specific stages or adjusting resource allocation per stage according to the pipeline configuration.
Concept of Domain Adaptation
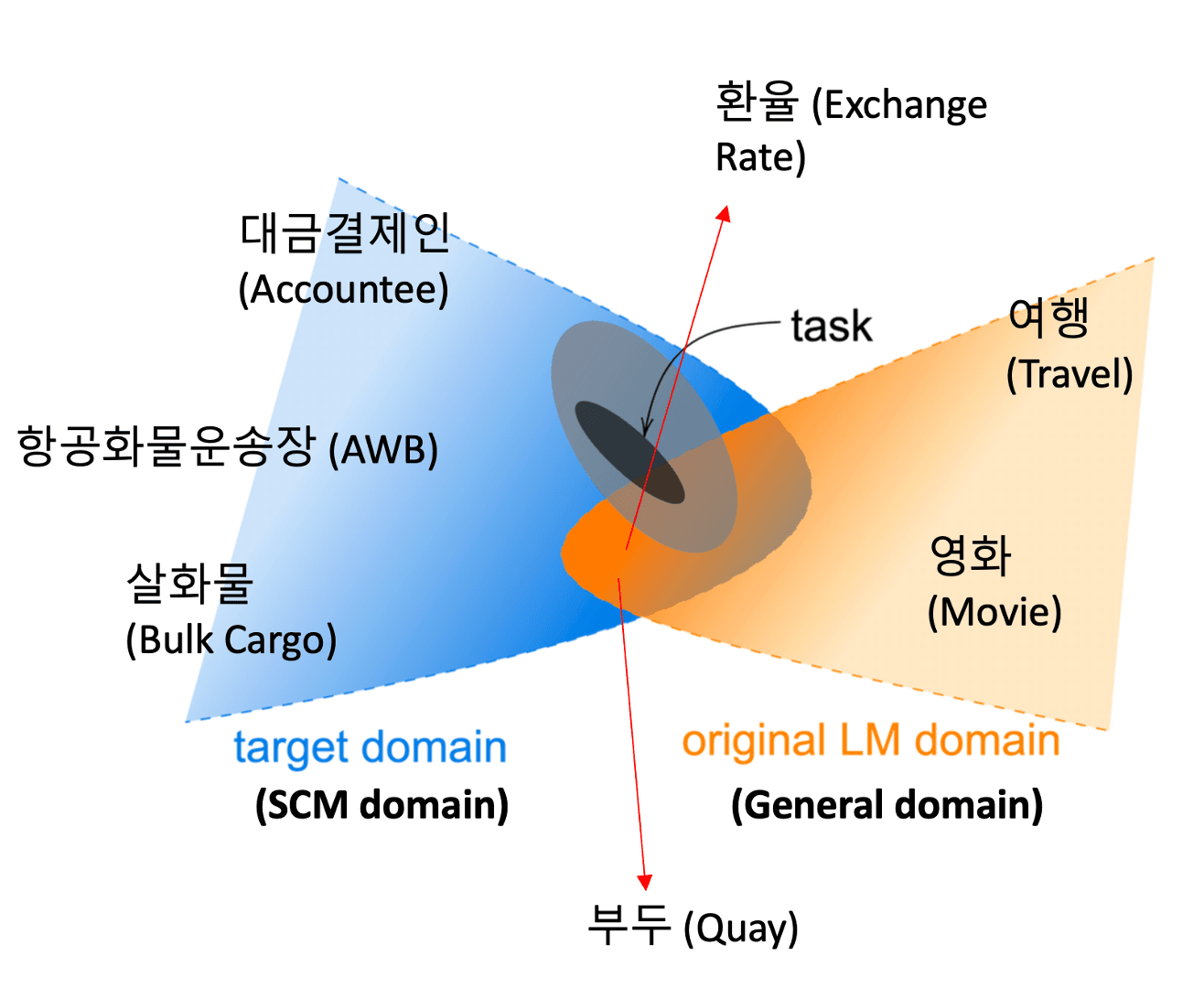
Before diving into model training, a process called Domain Adaptation is necessary. To briefly explain for those unfamiliar, Domain Adaptation refers to the process of refining a pretrained model to make it suitable for a specific domain. Most general-purpose language models we encounter today are not designed to possess expertise in specific fields. These models are typically trained using datasets from general domains to predict the next token effectively and then fine-tuned to fit overall usage directions.
However, when creating a model for use in a specialized domain, training with general datasets is insufficient. For instance, a model trained in a general domain can understand contexts like "This movie was amazing," but it may struggle with sentences in the legal domain, such as "The court ordered the seizure of the debtor's assets," due to the lack of learning of specific terms and expressions used in each domain. Similarly, if a Q/A task is given, implementing it with general data might not be possible. To properly handle a Q/A task, a language model must be fine-tuned with domain-specific datasets trained for the Q/A task. This fine-tuning process allows the model to better understand the nuances of the task and effectively respond to domain-specific questions from the user.
This article focuses on the process of developing a model specialized in Supply Chain Management (SCM) and trade domains. As shown in the above image, there is a significant difference between general domain terms like "movie" or "travel" and SCM-specific terms like "air waybill" or "payment manager." To bridge this gap, our goal today is to adjust the model using datasets from SCM and trade domains to enhance the model's understanding of these domains and accurately capture the context.
In summary, Domain Adaptation is essentially a process of overcoming the gaps between different domains, enabling the model to perform better in new contexts.
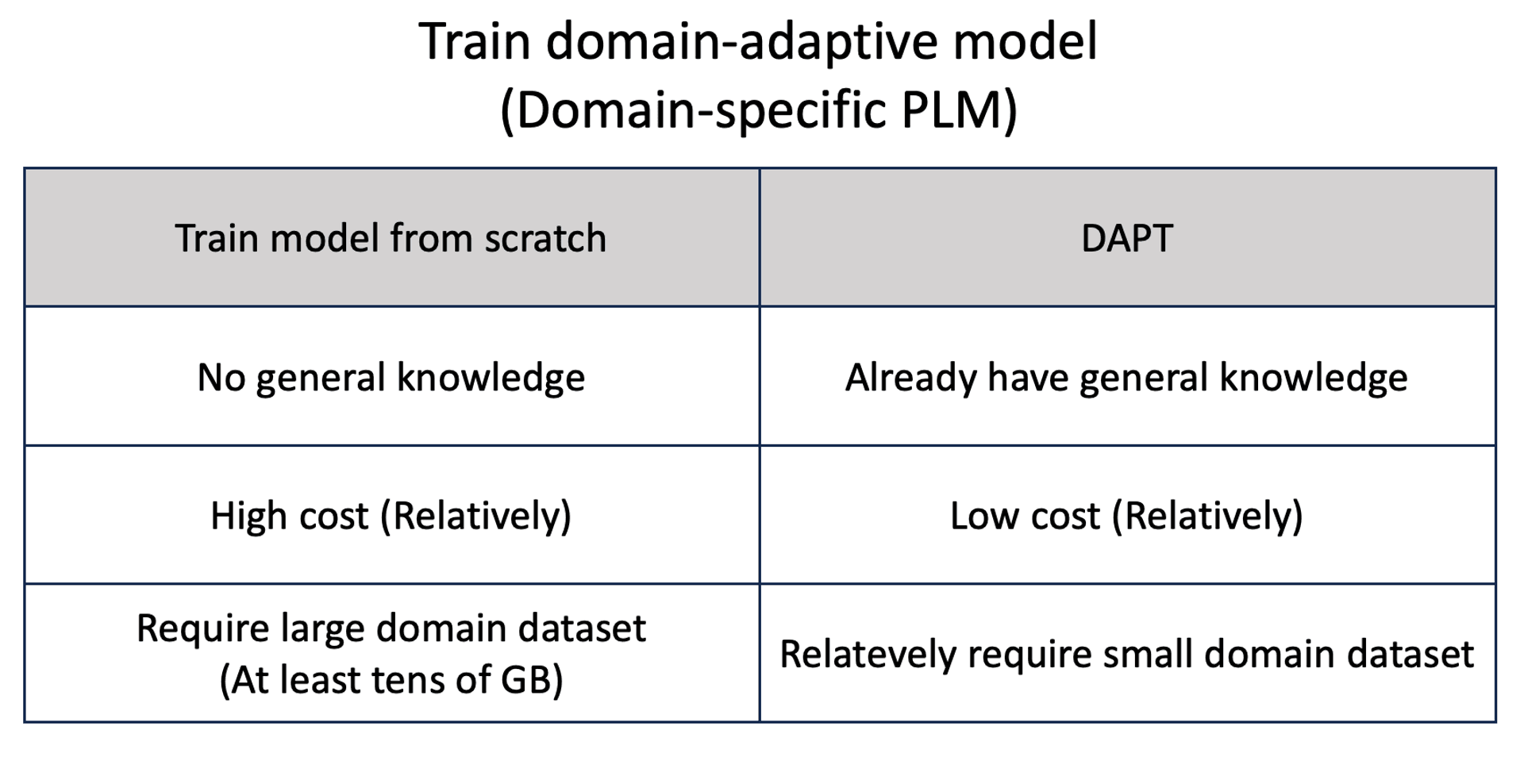
Train model from scratch vs DAPT
So, why not train the model from scratch using datasets from the specific domain? While this is possible, it comes with several limitations. Training a model from scratch with domain-specific datasets requires extensive data and training because the model lacks both general domain knowledge and domain-specific expertise. Collecting datasets for general domain deep learning is already challenging, but gathering high-quality, domain-specific data is even more difficult. Even if data is collected, preprocessing it to fit model training can be time-consuming and costly. Therefore, training a model from scratch is more suitable for companies with abundant domain-specific data and resources.What if you want to develop a domain-adaptive model but don't have access to vast datasets or sufficient resources? In such cases, Domain-Adaptive Pre-Training (DAPT) can be an effective approach. DAPT involves continual pretraining of a model that has already been extensively trained on general domains with domain-specific datasets to develop a specialized model. Since this method builds upon a model that already possesses knowledge of general domains, it requires relatively less cost and fewer datasets compared to training a model from scratch.
Development environment Setup
- Package Installation
pip install bitsandbytes==0.43.2 pip install deepspeed==0.14.4 pip install transformers==4.43.3 pip install accelerate==0.33.0 pip install flash-attn==1.0.5 pip install xforms==0.1.0 pip install datasets==2.20.0 pip install wandb pip install evaluate==0.4.2 pip install vertexai==1.60.0 pip install peft==0.12.0 pip install tokenizers==0.19.1 pip install sentencepiece==0.2.0 pip install trl==0.9.6 pip install bitsandbytes==0.43.2 pip install deepspeed==0.14.4 pip install transformers==4.43.3 pip install accelerate==0.33.0 pip install flash-attn==1.0.5 pip install xforms==0.1.0 pip install datasets==2.20.0 pip install wandb pip install evaluate==0.4.2 pip install vertexai==1.60.0 pip install peft==0.12.0 pip install tokenizers==0.19.1 pip install sentencepiece==0.2.0 pip install trl==0.9.6
- Import Modules
import os import json from datasets import load_from_disk, Dataset,load_dataset import torch from transformers import AutoTokenizer, AutoModelForCausalLM, Gemma2ForCausalLM, BitsAndBytesConfig, pipeline, TrainingArguments from peft import LoraConfig, get_peft_model import transformers from trl import SFTTrainer from dotenv import load_dotenv import wandb from huggingface_hub import login
Dataset preparation
The preparation of datasets should vary depending on the purpose of fine-tuning. In this article, since our goal is to train a model that can effectively respond to questions related to the trade domain, we decided to use datasets that we collected ourselves through web crawling. The datasets are categorized into three types: trade certification exam datasets, trade term-definition datasets, and trade lecture script datasets.
- Trade Certification Exam Data Set
질문: 다음 중 우리나라 대외무역법의 성격에 대한 설명으로 거리가 먼 것을 고르시오. 1. 우리나라에서 성립되고 이행되는 대외무역행위는 기본적으로 대외무역법을 적용한다. 2. 타 법에서 명시적으로 대외무역법의 적용을 배제하면 당해 법은 특별법으로서 대외무역법보다 우선 적용된다. 3. 대외무역법은 국내법으로서 국민의 국내 경제생활에 적용되는 법률이기 때문에 외국인이 국내에서 행하는 무역행위는 그 적용 대상이 아니다. 4. 관계 행정기관의 장은 해당 법률에 의한 물품의 수출·수입 요령 그 시행일 전에 지식경제부 장관이 통합하여 공고할 수 있도록 제출하여야 한다. 정답: 대외무역법은 국내법으로서 국민의 국내 경제생활에 적용되는 법률이기 때문에 외국인이 국내에서 행하는 무역행위는 그 적용 대상이 아니다. 질문: ...
- Trade Terms Definition Data Set
{
"term": "(계약 등을) 완전 무효화하다, 백지화하다, (처음부터) 없었던 것으로 하다(Rescind)",
"description": "계약을 파기, 무효화, 철회, 취소하는 것; 그렇지 않았음에도 불구하고 계약을 시작부터 무효인 것으로 선언하고 종결짓는 것."
}
- Trade Lecture Script Dataset
예전에는 전자상거래 셀러가 엑셀에다가 입력을 해서 수출신고 데이터를 업로드 해서 생성을 했잖아요 그리고 대량으로 전송하는 셀러는 api를 통해서 신고를 했습니다 그런데 그 수출신고 정보의 원천정보를 뭐냐면 쇼핑몰에서 제공하는 판매 주문정보입니다 그래서 그 쇼핑몰에 직접 저희가 연계를 해서 판매 주문 정보를 가져올 수 있게끔 새 서비스를 만들었어요 그래서 API 연계된 쇼핑몰들이 있는데 그게 현재 5개가 연결되어 있는데 쇼피 쇼피파이 라자다 라쿠텐 q10이 있고요 아마존하고 위치도 연계 예정에 있습니다 그래서 셀러는 ...
To create a model suitable for Q/A tasks, the datasets need to be converted into a question-and-answer format. The first dataset, the trade certification exam dataset, and the second dataset, the trade term-definition dataset, can be converted using simple code. However, upon examining the third dataset, the trade lecture script dataset, it appears challenging to directly convert the conversational data. In this case, an approach can be employed that uses large language models (LLMs) to extract Q/A pairs from the conversational scripts. The prompts and their results are as follows.
Prompt
summary: {summary}
Instruction: 다음 summary를 기반으로 질문과 해당 답변을 추출하세요.
출력 형식은 JSON으로, 각 질문과 답변을 객체로 묶어 'qa_pairs'라는 배열 안에 넣어야 합니다.
'questions' key에는 요약에서 도출된 질문 목록이 포함되어야 하며, 'answers' key에는 각 질문에 대한 해당 답변 목록이 포함되어야 합니다:
출력 예시:
{{"qa_pairs": [{{"question": "Question1", "answer": "Answer1"}},{{"question": "Question2", "answer": "Answer2"}},{{"question": "Question3", "answer": "Answer3"}},]...}}
Output:
Output
{"qa_pairs":
[
{"question": "전자상거래 무역 플랫폼은 어떤 서비스를 제공하나요?", "answer": "판매 주문 관리, 창고 관리, 수출입 통관, 배송 관리 등 전자상거래 업무를 위한 다양한 서비스를 제공합니다"},
{"question": "쇼핑몰과의 API 연계를 통해 무엇을 간소화할 수 있나요?", "answer": "판매 주문 정보를 자동으로 가져와 수출신고를 간소화할 수 있습니다"},
{"question": "창고 관리 기능은 어떤 것을 관리할 수 있나요?", "answer": "자가 창고, 위촉 창고, 해외 풀필먼트 센터 등을 관리할 수 있습니다"},
{"question": "수출입 통관 서비스는 어떤 기능을 제공하나요?", "answer": "미국 세관과 연동된 수출입 통관 서비스를 통해 미국 수출 시 수입 신고를 간편하게 처리할 수 있습니다"},
{"question": "배송 관리 기능은 어떤 서비스를 지원하나요?", "answer": "우체국 EMS, DHL 등 개별 배송과 함께 해외 현지 라스트 마일 배송까지 지원합니다"}
]
}
It looks like we are now ready to convert each dataset into a Q/A dataset using simple code. Below is the code that demonstrates how to convert each dataset into Q/A format.
import os
import json
import re
from datasets import Dataset, concatenate_datasets, load_from_disk
def replace_dot_number(text):
result = re.sub(r'\.(\d+)\.', r'. \1.', text)
return result
def read_json(path):
with open(path, 'r', encoding='utf-8') as f:
return json.load(f)
def write_json(data, path):
with open(path, 'w', encoding='utf-8') as f:
json.dump(data, f, ensure_ascii=False)
def dataset_maker(data:list) -> Dataset:
return Dataset.from_list(data)
def save_dataset(dataset, save_path):
dataset.save_to_disk(save_path)
def exam_qa_formatter():
data = []
root = 'dataset/exam_data'
for file in sorted(os.listdir(root)):
file_path = os.path.join(root, file)
content = read_json(file_path)['fixed_text']
question_list = content.split('질문:')[1:]
for question in question_list:
try:
question_and_options = replace_dot_number(question.split('정답:')[0]).strip()
answer = question.split('정답:')[1].strip()
data.append({"context": replace_dot_number(question), "question":question_and_options, "answer":answer})
except Exception as e:
pass
return data
def description_to_term_formattter(kor_term, eng_term, description):
context = f"{kor_term}: {description}"
question = f"설명: '{description}' 이 설명에 해당하는 무역 용어는 무엇인가요?"
answer = kor_term if eng_term is None else f"{kor_term}, {eng_term}"
return context, question, answer
def term_to_description(kor_term, eng_term, description):
context = f"{kor_term}: {description}"
question = f"'{kor_term}({eng_term})' 이라는 무역 용어는 어떤 의미인가요?" if eng_term is not None else f"'{kor_term}' 이라는 무역 용어는 어떤 의미인가요?"
answer = description
return context, question, answer
def term_qa_formatter():
data = []
root = 'dataset/term_data'
for file in os.listdir(root):
file_path = os.path.join(root, file)
term_set = read_json(file_path)
if file == 'terms_data_2.json':
term_set = [item for sublist in term_set for item in sublist]
for pair in term_set:
eng_term = pair.get('eng_term', None)
if 'term' in pair.keys():
kor_term = pair['term']
else:
kor_term = pair['kor_term']
description = pair['description']
context_1, question_1, answer_1 = description_to_term_formattter(kor_term, eng_term, description)
context_2, question_2, answer_2 = term_to_description(kor_term, eng_term, description)
data_1 = {"context": context_1, "question": question_1, "answer": answer_1}
data_2 = {"context": context_2, "question": question_2, "answer": answer_2}
data.append(data_1)
data.append(data_2)
return data
def transcript_qa_formatter():
data = []
root = 'dataset/transcript_data/success'
for file in sorted(os.listdir(root)):
file_path = os.path.join(root, file)
for line in open(file_path):
line = json.loads(line)
context = line['context']
output = line['json_output']
qa_pairs = json.loads(output)['qa_pairs']
for pair in qa_pairs:
question = pair['question']
answer = pair['answer']
if type(answer) == list:
answer = answer[0]
data.append({"context": context, "question": question, "answer": answer})
return data
###### Term dataset
{'context': 'APEC 경제위원회(Economic Committee (EC)): 개별위원회나 실무그룹이 추진하기 어려운 여러분야에 걸친 이슈에 대한 분석적 연구작업을 수행하기 위해 결성된 APEC 기구,',
'question': "설명: '개별위원회나 실무그룹이 추진하기 어려운 여러분야에 걸친 이슈에 대한 분석적 연구작업을 수행하기 위해 결성된 APEC 기구,' 이 설명에 해당하는 무역 용어는 무엇인가요?",
'answer': 'APEC 경제위원회(Economic Committee (EC))'}
###### Transcript dataset
{'context': '수입 신고는 일반적으로 입항 후에 하는 것이 원칙이며, 보세 구역에서 5부 10장을 작성하여 신고합니다',
'question': '수입 신고는 언제 하는 것이 원칙인가요?',
'answer': '수입 신고는 일반적으로 입항 후에 하는 것이 원칙입니다.'}
###### Exam dataset
{'context': ' 다음 중 우리나라 대외무역법의 성격에 대한 설명으로 거리가 먼 것을 고르시오. 1. 우리나라에서 성립되고 이행되는 대외무역행위는 기본적으로 대외무역법을 적용한다. 2. 타 법에서 명시적으로 대외무역법의 적용을 배제하면 당해 법은 특별법으로서 대외무역법보다 우선 적용된다. 3. 대외무역법은 국내법으로서 국민의 국내 경제생활에 적용되는 법률이기 때문에 외국인이 국내에서 행하는 무역행위는 그 적용 대상이 아니다. 4. 관계 행정기관의 장은 해당 법률에 의한 물품의 수출·수입 요령 그 시행일 전에 지식경제부 장관이 통합하여 공고할 수 있도록 제출하여야 한다.정답: 대외무역법은 국내법으로서 국민의 국내 경제생활에 적용되는 법률이기 때문에 외국인이 국내에서 행하는 무역행위는 그 적용 대상이 아니다.',
'question': '다음 중 우리나라 대외무역법의 성격에 대한 설명으로 거리가 먼 것을 고르시오. 1. 우리나라에서 성립되고 이행되는 대외무역행위는 기본적으로 대외무역법을 적용한다. 2. 타 법에서 명시적으로 대외무역법의 적용을 배제하면 당해 법은 특별법으로서 대외무역법보다 우선 적용된다. 3. 대외무역법은 국내법으로서 국민의 국내 경제생활에 적용되는 법률이기 때문에 외국인이 국내에서 행하는 무역행위는 그 적용 대상이 아니다. 4. 관계 행정기관의 장은 해당 법률에 의한 물품의 수출·수입 요령 그 시행일 전에 지식경제부 장관이 통합하여 공고할 수 있도록 제출하여야 한다.',
'answer': '대외무역법은 국내법으로서 국민의 국내 경제생활에 적용되는 법률이기 때문에 외국인이 국내에서 행하는 무역행위는 그 적용 대상이 아니다.'}
# Exam dataset
Dataset({
features: ['context', 'question', 'answer'],
num_rows: 1430
})
# Term dataset
Dataset({
features: ['context', 'question', 'answer'],
num_rows: 15678
})
# Transcript dataset
Dataset({
features: ['context', 'question', 'answer'],
num_rows: 8885
})
# Concatenated dataset
Dataset({
features: ['context', 'question', 'answer'],
num_rows: 25993
})
The combined dataset (training dataset) with the Q/A format is as above. About 26,000 Q/A pairs are expected to be used for training.
Now, the dataset for fine-tuning is ready. Let’s check how this dataset is actually fed into the model.
<bos><start_of_turn>user
Write a hello world program<end_of_turn>
<start_of_turn>model
On the Huggingface website, you can find the model card for gemma-2-2b-it, which includes information on the chat template format and the definition of the model's prompt format (gemma-2-2b-it). This means that to ask questions to gemma, you need to create a prompt in a format that the model can understand.
The start of the conversation is marked with <start_of_turn>, and the end of the conversation is marked with <end_of_turn>. The speakers are specified as the user and the model. Therefore, when asking a question to the model, the prompt should follow this format.
def formatting_func(example):
prompt_list = []
for i in range(len(example['question'])):
prompt_list.append("""<bos><start_of_turn>user
다음 질문에 대답해주세요:
{}<end_of_turn>
<start_of_turn>model
{}<end_of_turn><eos>""".format(example['question'][i], example['answer'][i]))
return prompt_list
This document focuses on training the model using the Q/A dataset, so the approach will be to train the model in the manner of "for this type of question, respond in this way." Considering the previously mentioned chat template, you can write code in the format described above.
At this point, even if tokens are not explicitly included in the chat template, the model may attempt to generate more content beyond the delimiter. To ensure the model provides only an answer and then ends its turn, an <eos> token is added.
In actual training, examples like the one above will be used as input. Now, the dataset preparation for training is complete.
# Training
The training code is very simple. We use SFTTrainer, and as the base model, we use the gemma-2-2b-it model, which has been continually pretrained on SCM & trade datasets.
```python
model_id = "google/gemma-2-2b-it"
output_dir = 'QA_finetune/gemma-2-2b-it-lora128'
tokenizer = AutoTokenizer.from_pretrained(model_id, token=access_token)
model = AutoModelForCausalLM.from_pretrained(
# "google/gemma-2-2b-it",
"yonggeun/gemma-2-2b-it-lora128-merged",
device_map="auto",
torch_dtype=torch.bfloat16,
token=access_token,
attn_implementation="eager", # attn_implementation,
cache_dir="./models/models",
)
def formatting_func(example):
prompt_list = []
for i in range(len(example['question'])):
prompt_list.append("""<bos><start_of_turn>user
다음 질문에 대답해주세요:
{}<end_of_turn>
<start_of_turn>model
{}<end_of_turn><eos>""".format(example['question'][i], example['answer'][i]))
return prompt_list
def train(data):
valid_set = data["test"]
valid_set.save_to_disk('QA_finetune/valid_set/gemma-2-2b-it-lora128')
lora_config = LoraConfig(
r=256,
lora_alpha=32,
lora_dropout=0.05,
bias="none",
target_modules=["q_proj", "o_proj", "k_proj", "v_proj", "gate_proj", "up_proj", "down_proj"],
task_type="CAUSAL_LM",
)
training_args = TrainingArguments(
per_device_train_batch_size=2,
warmup_steps=2,
logging_steps=1,
gradient_accumulation_steps=4,
# num_train_epochs=3,
num_train_epochs=3,
learning_rate=2e-4,
save_steps=100,
fp16=False,
bf16=True,
output_dir=output_dir,
push_to_hub=True,
report_to="wandb"
)
trainer = SFTTrainer(
model=model,
tokenizer=tokenizer,
train_dataset=data['train'],
args=training_args,
formatting_func=formatting_func,
peft_config=lora_config,
max_seq_length=max_length,
packing= False,
)
model.config.use_cache = False
print("Training...")
trainer.train()
print("Training done!")
Evaluation
Once the training is successfully completed, it is essential to evaluate the model's performance. This article focuses on evaluating Question Answering performance in a specific domain, which required different metrics than those typically used for benchmarking general models. In this article, the model was evaluated using SemScore and Truthfulness.
SemScore: An evaluation method based on the semantic textual similarity between the target response and the model's response. (SemScore)
Evaluating Truthfulness: This method measures truthfulness on a scale of 1 to 5 by providing the model's response and the target answer to an LLM. (Truthfulness)
Fasttrack pipeline
Now, let’s create a pipeline in FastTrack that will be used for model training. A pipeline is a unit of work used in FastTrack. Each pipeline can be represented as a collection of tasks, which are the smallest executable units. Multiple tasks within a single pipeline can have dependencies on each other, and their sequential execution is ensured based on these dependencies.
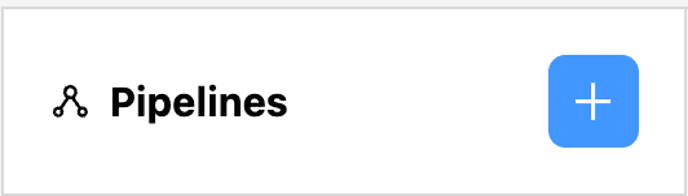
Create Pipeline
In the image above, find the blue '+' button to create a new pipeline.
When creating a pipeline, you can choose the pipeline’s name and description, the location of the data repository to be used, and the environment variables that will be commonly applied in the pipeline. After entering the necessary information, click the "Save" button at the bottom to create the pipeline.
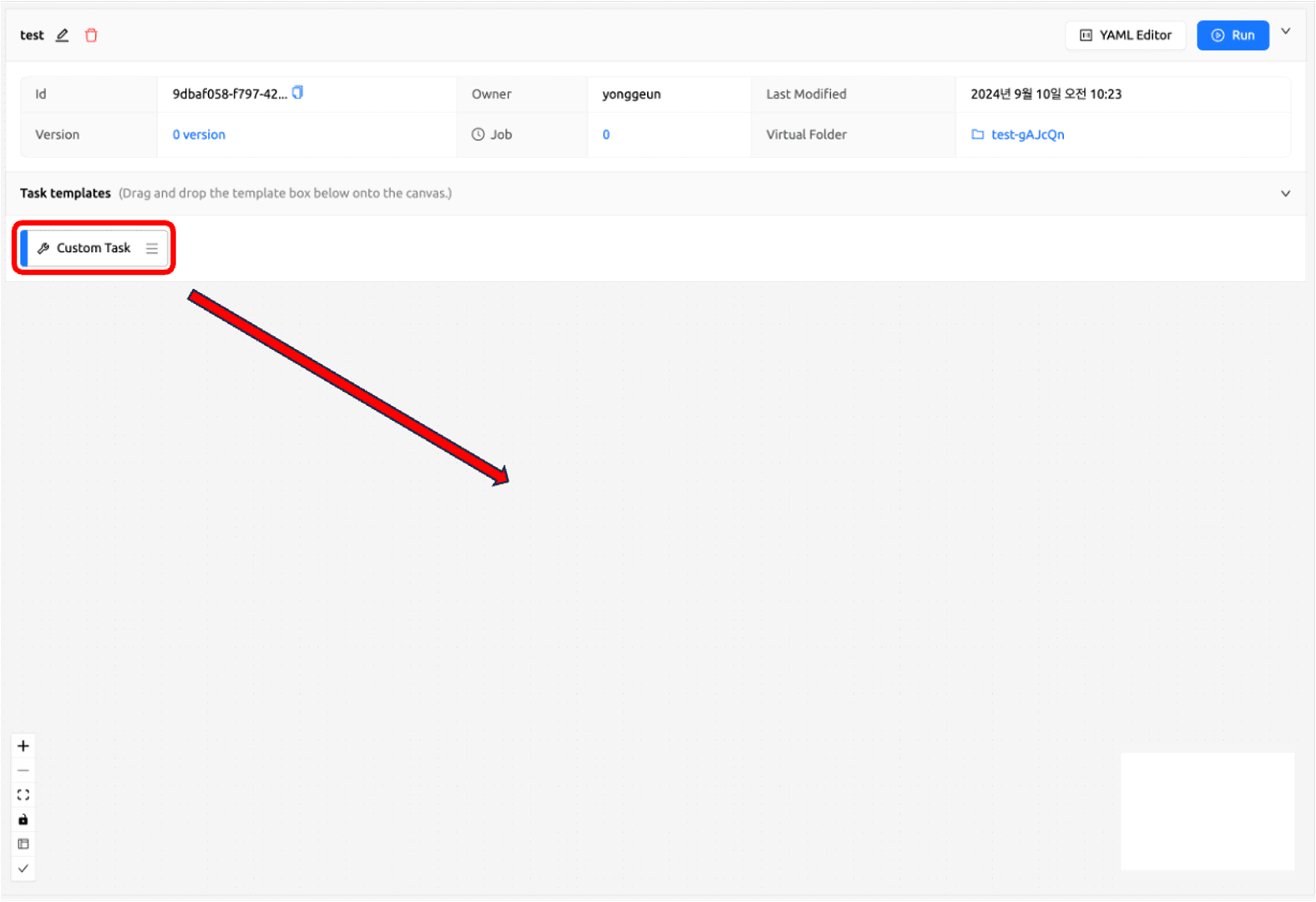
Drag and create task
Once a new pipeline is created, you can add a new task to the task template. Click on the "Custom Task" and drag it into the workspace below to create a new task.
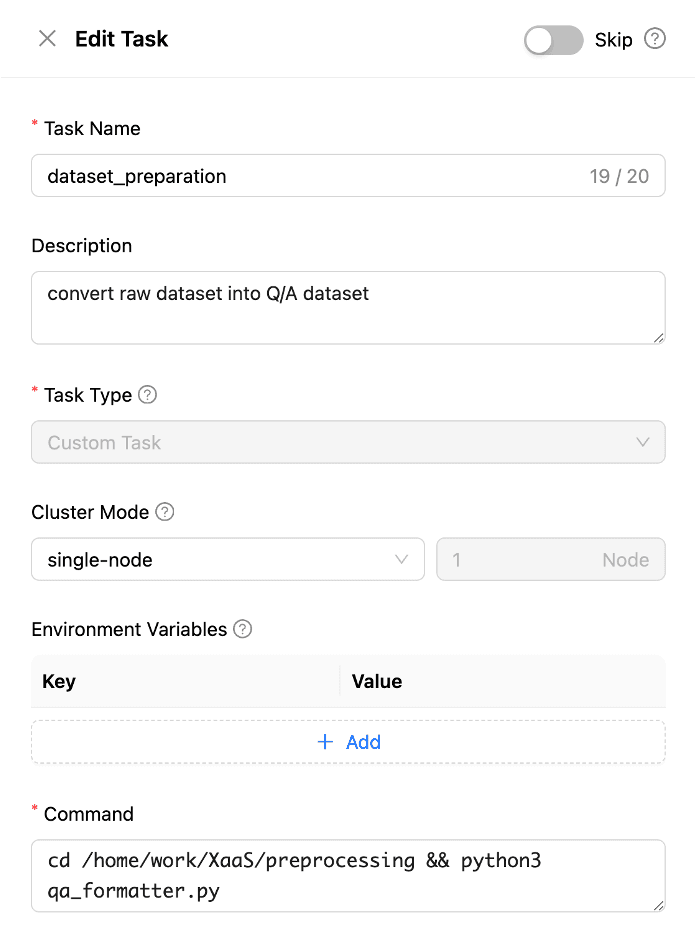
Enter information
When creating a task, you need to enter the information required for task execution, as shown above. Write the task name and description clearly, and choose between a single node or multiple nodes. In this document, we will perform training on a single node, so we will select a single node.
Next, you need to write the command. The command essentially runs the session. Make sure to specify the directory of the mounted V-folder correctly so that the script runs without errors. Most of the packages required for training are already installed in the session, but if additional packages need to be installed or there are version issues, you may need to reinstall the packages. In such cases, you can specify the required packages in the requirements.txt file, install them, and then run the other scripts.
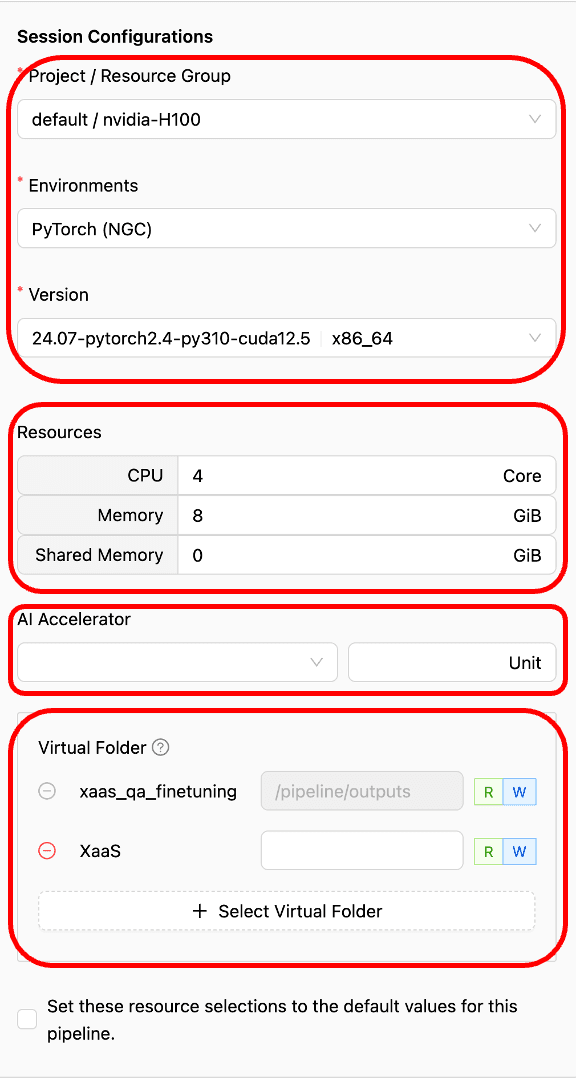
Resource configuration
Next are the settings for the session, resources, and V-folder.
Although the code in this article is written based on PyTorch, you can also choose other environments like TensorFlow, Triton server, etc.
One of the advantages of FastTrack is its ability to utilize resources as efficiently as possible. Even within a single resource group, resources can be divided among multiple sessions, maximizing the resource utilization rate.
For dataset preparation, GPU computation is not required, so it is acceptable not to allocate GPU resources. This allows you to run the code with minimal resources and allocate GPU resources to other sessions during this time, preventing GPU resources from remaining idle. Furthermore, if parallel model training is needed (e.g., when 10 GPUs are available and each training session requires 5 GPUs), you can train models in parallel. This approach helps reduce resource wastage and shortens training time.
Select the V-folder where the prepared dataset and training code are correctly located.
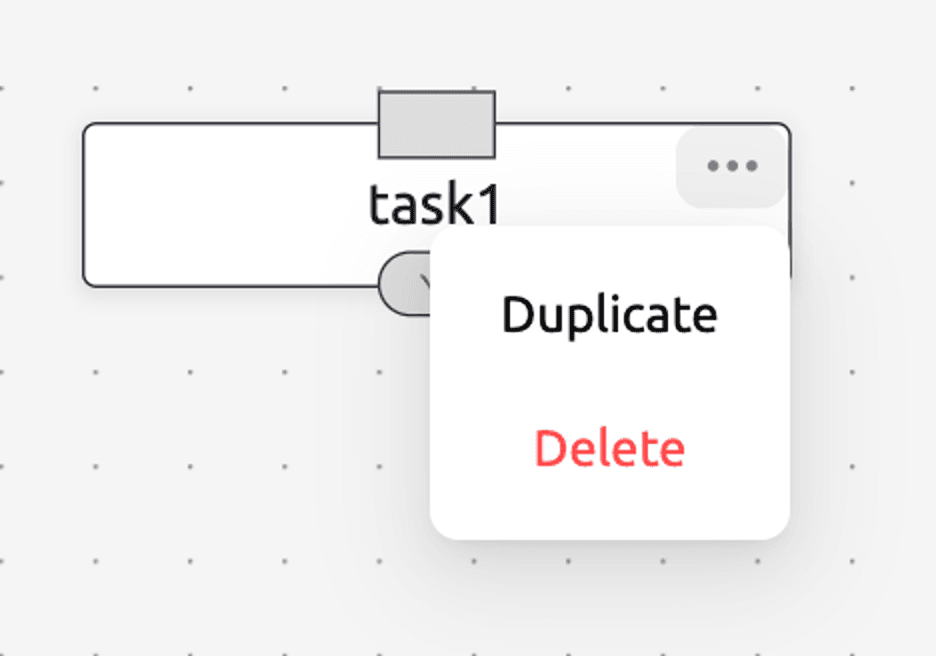
Duplicate or delete task
By clicking the meatball menu icon (⋯) at the top right corner of the task block, you can duplicate or delete the created task.
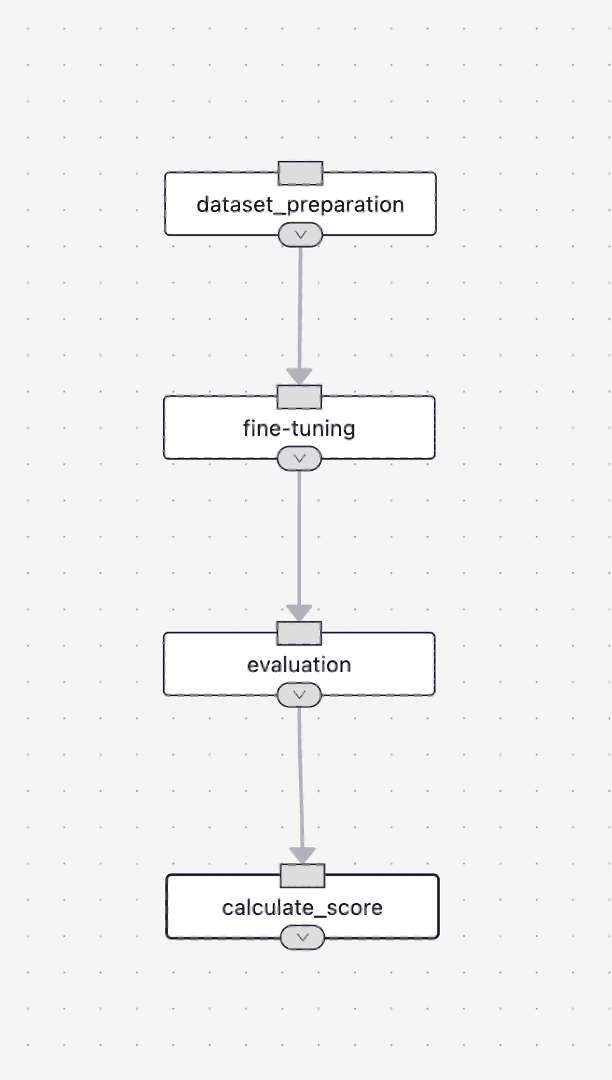
In FastTrack, you can set the order between multiple created tasks like this. This process involves adding dependencies between tasks. In some cases, you can set the next task to run only after several tasks are completed. In such cases, the next task will not proceed until all dependent tasks are finished. The completed example is shown above. In this article, we will proceed in the order of dataset preparation - fine-tuning - evaluation.
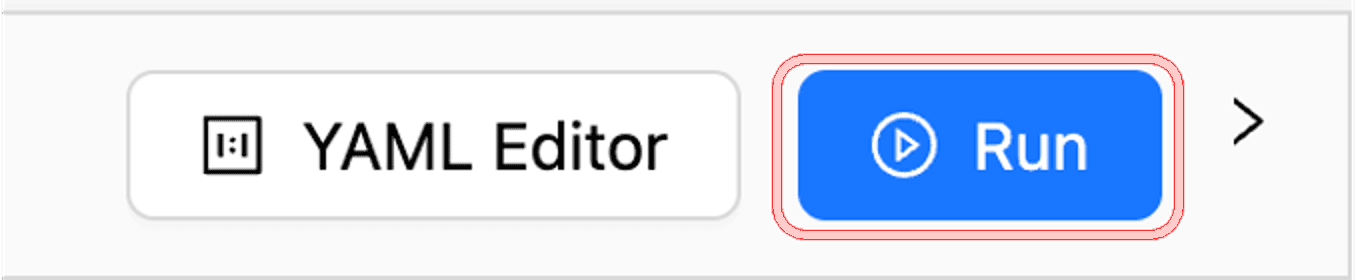
If each task is defined correctly, click "Run" to execute the pipeline.
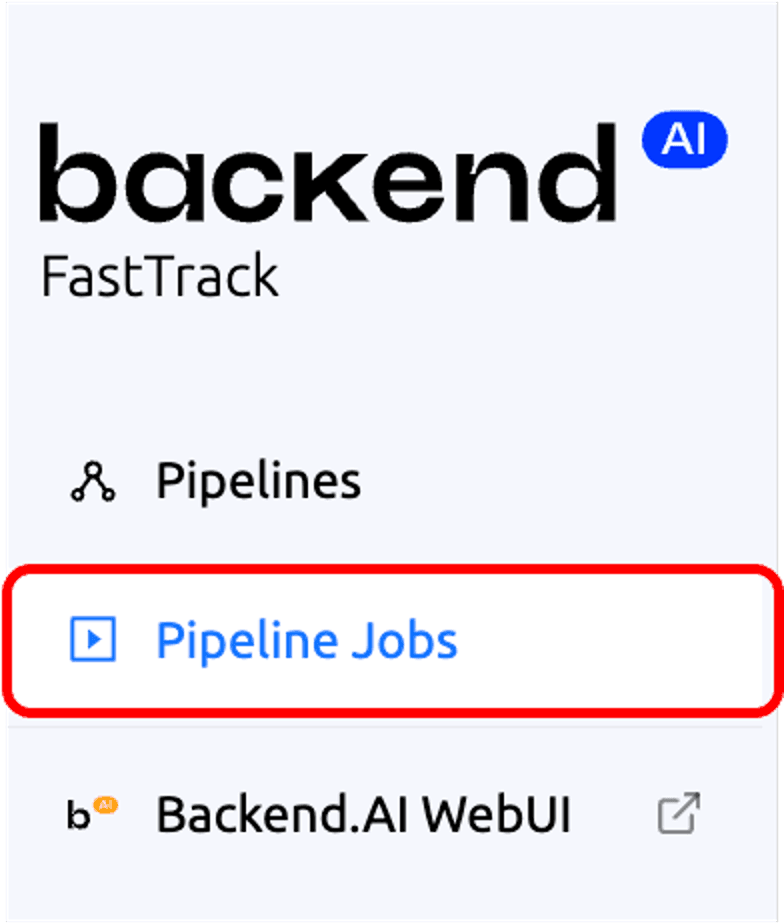
On the left side of the FastTrack screen, you can see the pipelines you created. By clicking on them, you can monitor the currently running tasks and previously executed tasks in the pipeline task session.
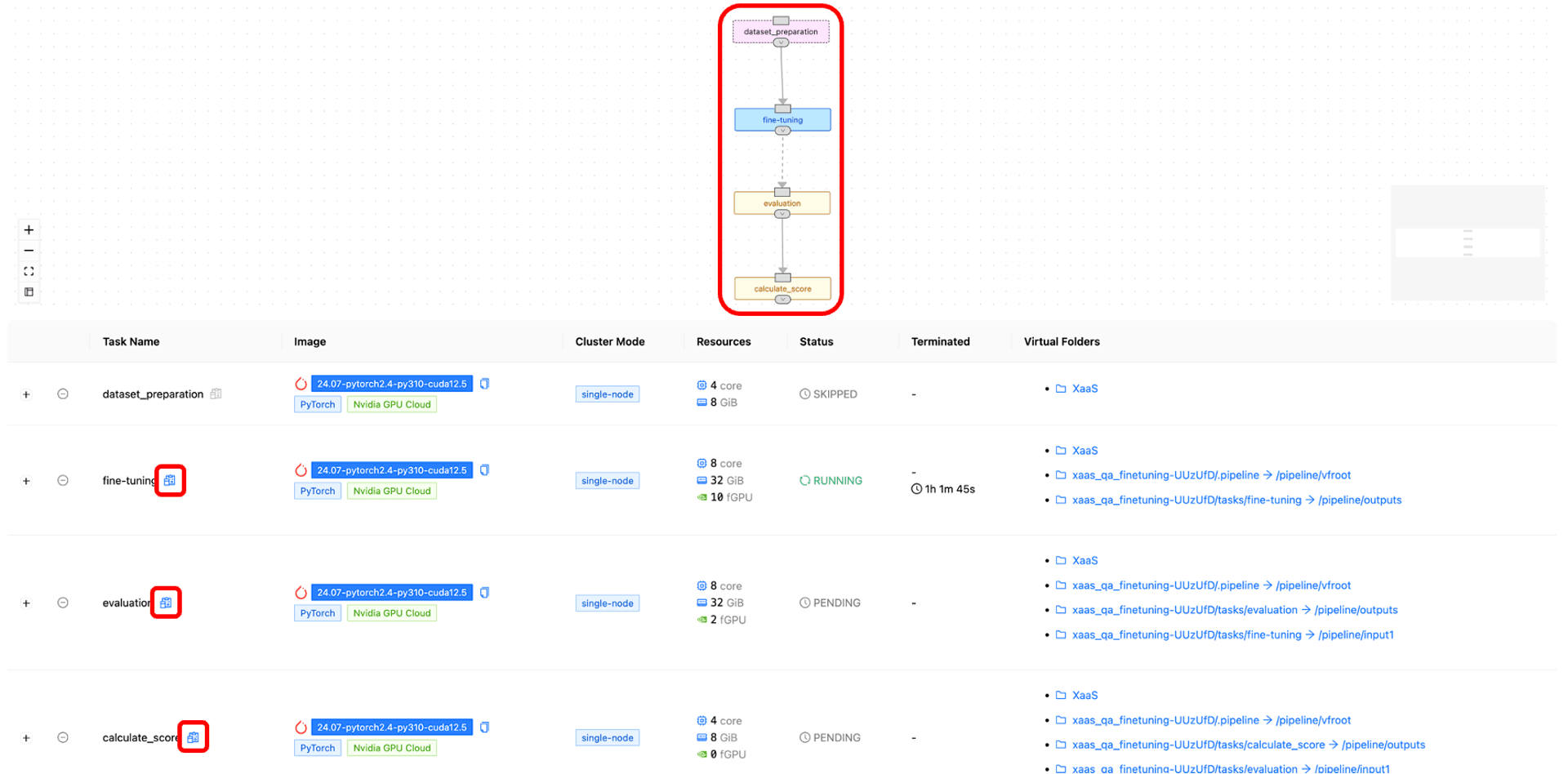
Monitoring jobs
You can monitor the tasks through a screen like the one above. Each task proceeds in the specified order; once a previous task is completed, resources are allocated to start the session for the next task, and when the task is done, the session is terminated. There is also an option to skip tasks if needed. For example, in the image above, you can see that the fine-tuning task is running after skipping the dataset preparation task.
Skipped tasks are shown in pink, running tasks are in light blue, and tasks scheduled to run are in yellow.
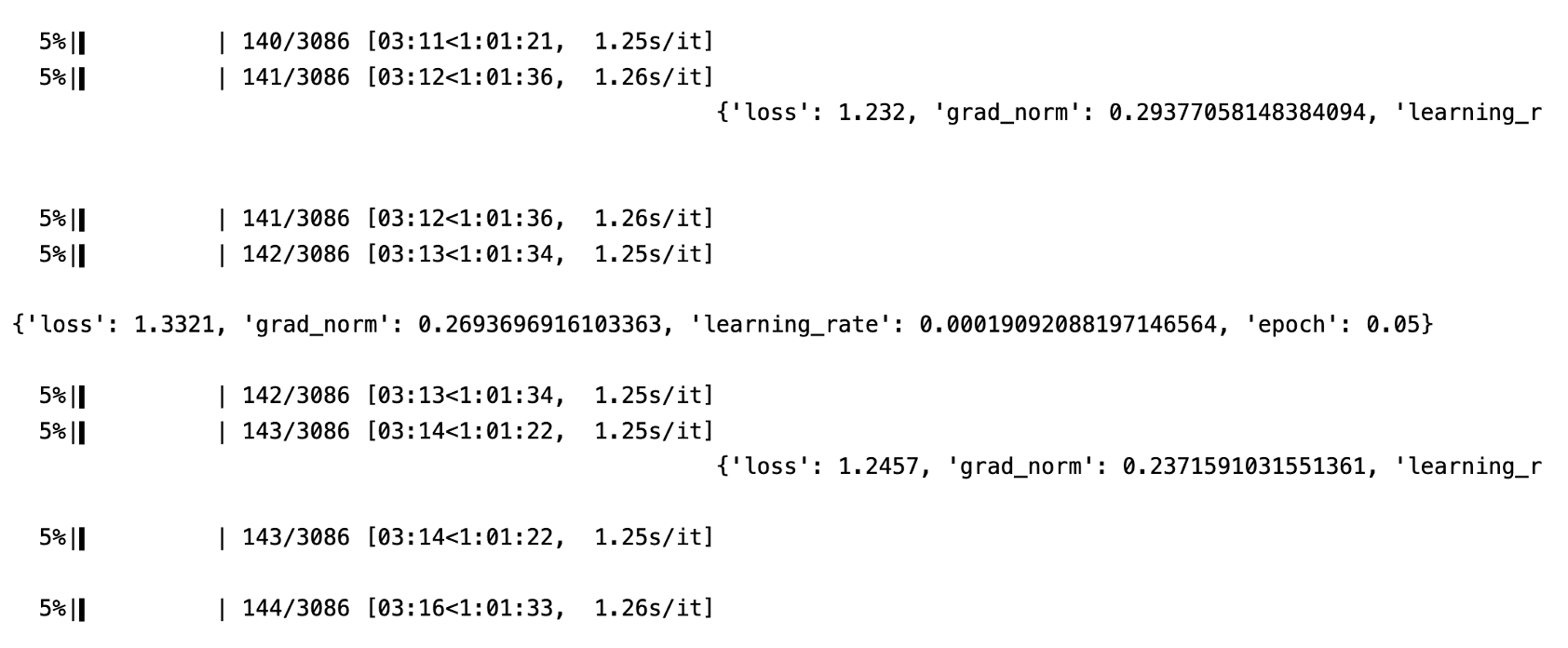
Log checking
By clicking the blue button next to each task's name, highlighted with a red square, you can check the logs of each task. This allows you to directly monitor the training progress. The logs appear the same as they would in a terminal, as shown in the screen above, allowing you to verify that the training is progressing correctly.
Once the pipeline execution is successfully completed, you can check the results. In this document, the evaluation results are plotted and saved as /home/work/XaaS/train/QA_finetune/truthfulness_result.png.
(Backend.AI's V-folder has a default directory structure of /home/work/~.)
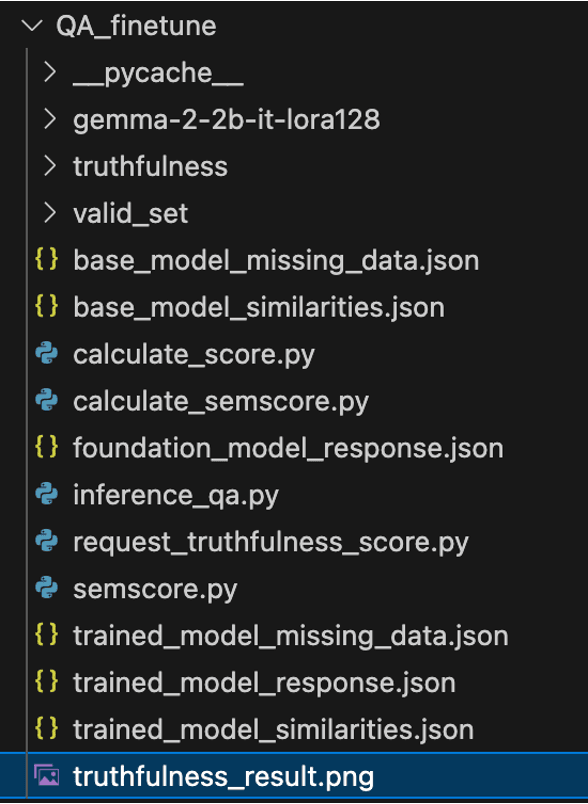
After training is complete, the result image is generated at the specified path.
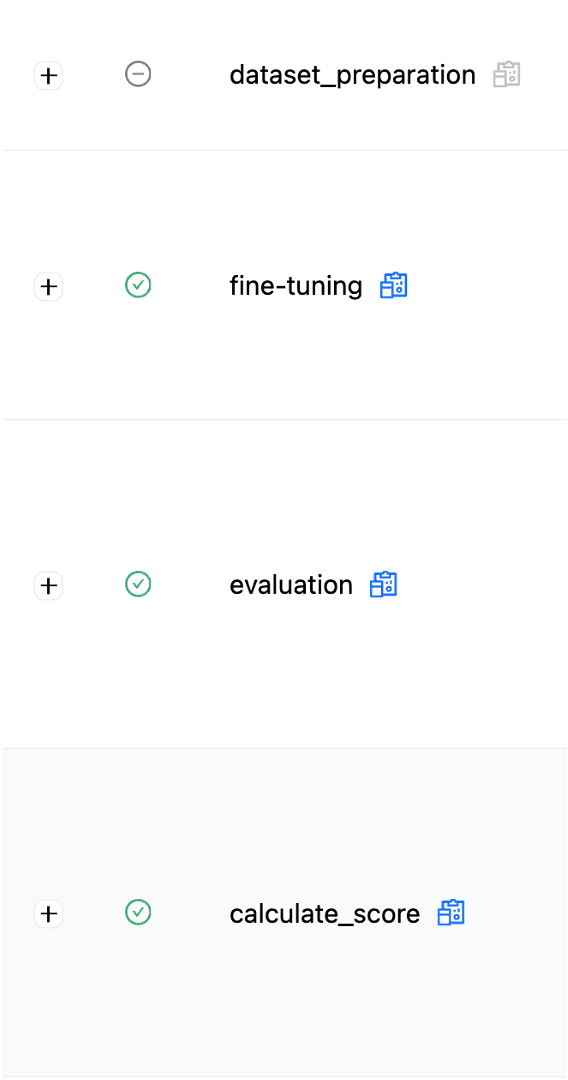
Result checking
As shown above, you can see the successful execution of the pipeline by checking to the left of each task name.
Result
Now, let’s compare the results of the fine-tuned model with the base gemma-2-2b-it model.
-
SemScore (Semantic text similarity between target response and model response, 1.00 is the best)
Base Model Trained Model 0.62 0.77
The SemScore of the trained model has increased (0.62 -> 0.77). This result indicates that the trained model can generate outputs that are more semantically similar to the target responses. In other words, the trained model has improved in generating responses that are closer to the intended target responses and more semantically consistent. As a result, the overall performance and reliability of the trained model have significantly improved.
-
Truthfulness The trained model shows a trend of increasing high-score cases and decreasing low-score cases. Low scores (1, 2 points) decreased (1,111 -> 777), while high scores (4, 5 points) increased (108 -> 376). This indicates that the model's ability to identify domain information closer to the truth has improved, showing that the training was effective.
Truthfulness result
Conclusion
In this article, we built a pipeline to train a model specialized in a specific domain using FastTrack, the MLOps platform of Backend.AI.
Even though we utilized only some of FastTrack’s features, it allowed us to flexibly manage resources, freely configure tasks, reduce training time, and improve resource utilization. Moreover, we were able to train models stably in independent execution environments and monitor the execution information of pipeline jobs, enabling us to track resource usage and execution counts for each pipeline during training.
In addition to the contents covered in this article, FastTrack supports a variety of additional features such as scheduling and parallel model training. For more information about other features of FastTrack, you can refer to the blog posts written by Kang Ji-hyun and Kang Jung-seok, linked below.
Although we did not fully utilize all of FastTrack's features, its flexible resource management and free task configuration helped shorten training time and increase resource utilization rates. Furthermore, it provided a stable training environment and allowed us to monitor resource usage and execution frequency within each pipeline through pipeline job execution information. FastTrack also supports many other functionalities such as scheduling and parallel model training. You can find more information about FastTrack in the documents below.